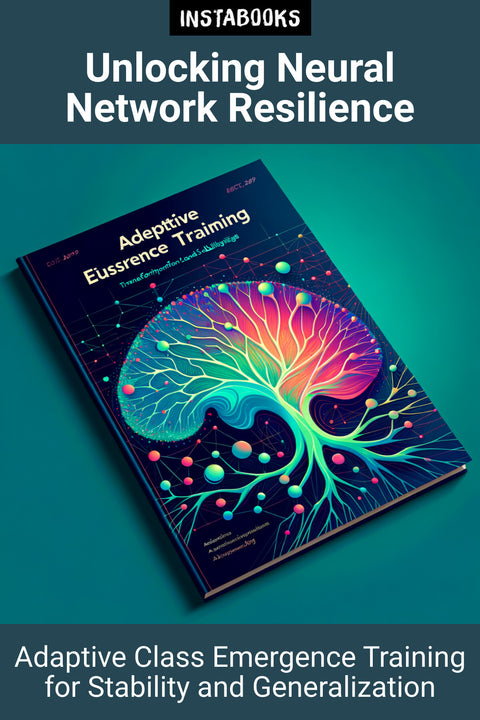
Instabooks AI (AI Author)
Unlocking Neural Network Resilience
Adaptive Class Emergence Training for Stability and Generalization
Premium AI Book - 200+ pages



Discover the Future of Neural Network Training with ACET
Adaptive Class Emergence Training (ACET) is revolutionizing the world of neural networks by enhancing stability and generalization through innovative techniques. This book serves as your definitive guide to understanding and implementing ACET in your AI projects, delivering insights into Progressive Target Evolution and Equilibrium-Based Training. Dive deep into the intricacies and benefits of ACET, where you will learn how this method transforms the traditional paradigms of neural network training.Explore Key Concepts and Methodologies
This comprehensive guide delves into traditional training methods and their limitations. By comparing ACET’s cutting-edge approach that employs Progressive Target Evolution, you'll understand its advantage in gradually transitioning target outputs, allowing networks to adapt smoothly to complex classification tasks. The book also explores Equilibrium-Based Training, inspired by structural equilibrium concepts from finite element analysis, enhancing network stability to prevent overfitting.Real-World Validation and Practical Applications
Uncover the results from extensive experiments conducted across diverse datasets, both synthetic and real-world. Witness how ACET provides faster convergence, improved accuracy, and superior generalization, particularly in high-complexity data scenarios. Discover practical applications where ACET can substantially impact performance, from image recognition to natural language processing, extending neural networks' robustness and reliability in real-world settings.A Look into the Future of Neural Network Training
Gain insights into the potential future directions of ACET as it integrates with other advanced methods to optimize neural network training. Explore how scalability challenges can be addressed, ensuring efficiency even with large datasets. Realize the potential for practical implementation of ACET across various industries, considering specific domain requirements and constraints to unleash the full potential of neural networks in your projects.Join the New Era of Stable and Generalized AI Models
With 'Unlocking Neural Network Resilience,' you are not just learning about a theoretical approach; you are stepping into the future of AI development. The book’s thorough research ensures that you are equipped with the knowledge and tools to harness ACET for enhanced neural network training, opening up new possibilities in AI stabilization and generalization. Embark on this transformative journey and redefine your approach to neural network challenges.Table of Contents
1. Understanding Adaptive Class Emergence Training- Introduction to ACET
- Traditional Training Methods
- ACET vs Traditional Approaches
2. Progressive Target Evolution Explained
- Concept and Principles
- Transition Strategies
- Benefits over Static Targets
3. Equilibrium-Based Training Techniques
- Structural Equilibrium Inspirations
- Implementing Equilibrium
- Avoiding Overfitting
4. Validation Through Experiments
- Synthetic Dataset Studies
- Real-World Dataset Applications
- Comparative Results Analysis
5. Applications in AI and Machine Learning
- Image Recognition Advances
- Natural Language Processing Applications
- Broader AI Implications
6. Addressing Scalability Challenges
- Large Dataset Solutions
- Computational Efficiency
- Future Scalability Research
7. Integrating ACET with Existing Methods
- Complementary Techniques
- Case Studies
- Hybrid Training Models
8. Exploring Real-World Implementations
- Domain-Specific Applications
- Deployment Strategies
- Overcoming Practical Constraints
9. Future Directions in Neural Network Training
- Emerging Trends
- Research Opportunities
- Long-Term Implications
10. Key Takeaways and Best Practices
- Practical Integration Tips
- Common Pitfalls Avoided
- Maximizing ACET Benefits
11. Case Studies and Success Stories
- Innovative Use Cases
- Performance Metrics
- Learning from Leaders
12. Conclusion and Call to Action
- Recap of Core Concepts
- Future Learning Pathways
- Embracing AI Evolution
Target Audience
This book is written for data scientists, AI researchers, and machine learning practitioners eager to advance their understanding of neural network training methodologies and those interested in cutting-edge developments in AI stability and generalization.
Key Takeaways
- Understanding of Adaptive Class Emergence Training (ACET) and its principles.
- Insights into Progressive Target Evolution and its implementation.
- Knowledge of Equilibrium-Based Training and its benefits in neural network stability.
- Ability to apply ACET in practical, real-world AI scenarios.
- Awareness of the future potential and integration of ACET with other training methods.
- Strategies for handling high-complexity data with improved generalization.
How This Book Was Generated
This book is the result of our advanced AI text generator, meticulously crafted to deliver not just information but meaningful insights. By leveraging our AI book generator, cutting-edge models, and real-time research, we ensure each page reflects the most current and reliable knowledge. Our AI processes vast data with unmatched precision, producing over 200 pages of coherent, authoritative content. This isn’t just a collection of facts—it’s a thoughtfully crafted narrative, shaped by our technology, that engages the mind and resonates with the reader, offering a deep, trustworthy exploration of the subject.
Satisfaction Guaranteed: Try It Risk-Free
We invite you to try it out for yourself, backed by our no-questions-asked money-back guarantee. If you're not completely satisfied, we'll refund your purchase—no strings attached.